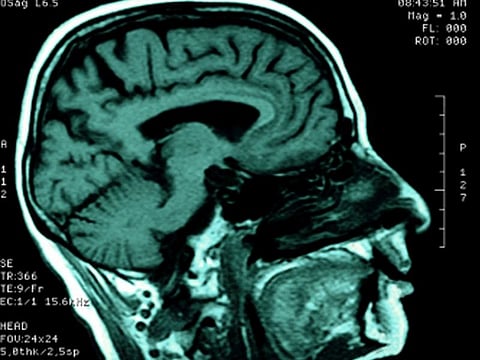
TUESDAY, Oct. 11, 2016 (HealthDay News) -- A radiomic-based approach can be used to generate a prediction model for stratifying treatment outcome among patients with recurrent glioblastoma prior to bevacizumab treatment, according to a study published online Oct. 10 in Clinical Cancer Research.
Philipp Kickingereder, M.D., from the University of Heidelberg Medical Center in Germany, and colleagues automatically extracted and analyzed 4,842 quantitative magnetic resonance imaging features from the multiparametric tumors of 172 patients with recurrent glioblastoma prior to bevacizumab treatment (allocated in a 2:1 ratio to a discovery and validation set). Radiomic features of patients in the discovery set were subjected to a supervised principal component (superpc) analysis leveraging a high throughput approach in order to develop a prediction model for stratifying treatment outcome to antiangiogenic therapy (progression-free [PFS] and overall survival [OS]).
The researchers found that patients in the discovery set were stratified into low- or high-risk groups for PFS and OS based on the superpc predictor (hazard ratios, 1.60 and 2.14, respectively). The stratification was validated in the validation set (hazard ratios, 1.85 and 2.60, respectively).
"Our radiomic-based superpc signature emerges as a putative imaging biomarker for the identification of patients who may derive the most benefit from antiangiogenic therapy, advances the knowledge in the noninvasive characterization of brain tumors, and stresses the role of radiomics as a novel tool for improving decision support in cancer treatment at low cost," the authors write.
One author disclosed financial ties to Genentech and Roche; Genentech manufactures bevacizumab.