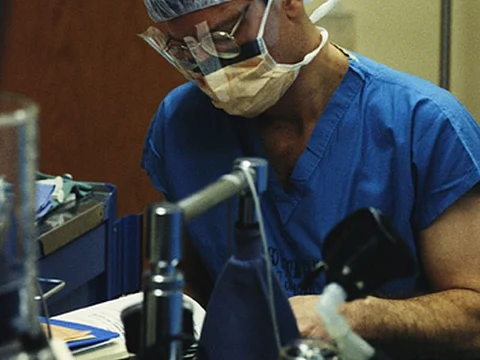
THURSDAY, Sept. 27, 2018 (HealthDay News) -- Machine learning of toxological big data can predict the toxicity of chemicals, and may be more reliable than animal testing, according to a study published in the September issue of Toxicological Sciences.
Noting that the probability that an OECD guideline animal test would output the same result in a repeat test was 78 to 96 percent, with sensitivity of 50 to 87 percent, Tom Luechtefeld, M.D., from the Johns Hopkins University Bloomberg School of Public Health in Baltimore, and colleagues used an expanded database with more than 866,000 chemical properties/hazards as training data. Read-across structure activity relationship (RASAR) models were constructed using binary fingerprints and Jaccard distance to define chemical similarity. This similarity metric was used to construct a large chemical similarity adjacency matrix, which was used to derive feature vectors for supervised learning. Results were demonstrated on nine health hazards from a "simple" and a "data fusion" RASAR.
The researchers found that the simple RASAR models achieved 70 to 80 percent balanced accuracies in cross-validation, with constraints on tested compounds. Balanced accuracies were in the 80 to 95 percent range across nine health hazards in cross-validation of data fusion RASARs, with no constraints on tested compounds.
"These results are a real eye-opener -- they suggest that we can replace many animal tests with computer-based prediction and get more reliable results," a coauthor said in a statement.
Several authors disclosed financial ties to Underwriters Laboratories; two authors created ToxTrack LLC to develop computational tools.
Abstract/Full Text (subscription or payment may be required)